File size: 12,547 Bytes
e090e58 71b8b06 e090e58 a5da72c e090e58 68b9739 286159b e090e58 9dba27c e090e58 9dba27c e090e58 e3fc972 e090e58 9dba27c e090e58 9dba27c e3fc972 9dba27c e3fc972 9dba27c e3fc972 9dba27c e3fc972 9dba27c e3fc972 9dba27c e3fc972 9dba27c e3fc972 9dba27c e3fc972 9dba27c e3fc972 9dba27c e3fc972 9dba27c e3fc972 9dba27c e090e58 9dba27c e090e58 9dba27c e090e58 9dba27c e090e58 9dba27c e3fc972 e090e58 9dba27c e090e58 9dba27c e090e58 9dba27c e090e58 cd389b1 e090e58 9dba27c e090e58 a5da72c e090e58 9dba27c 30640f7 9dba27c 30640f7 e090e58 30640f7 9dba27c a5da72c e090e58 30640f7 9dba27c 30640f7 9dba27c 5cb0eba 9dba27c 30640f7 e090e58 30640f7 9dba27c e090e58 9dba27c e090e58 9dba27c e090e58 9dba27c e090e58 cd389b1 673a675 a5da72c 673a675 a5da72c 673a675 a5da72c 673a675 a5da72c 673a675 a5da72c 673a675 a5da72c 673a675 a5da72c 673a675 a5da72c 673a675 a5da72c 673a675 a5da72c 673a675 a5da72c 673a675 a5da72c 673a675 a5da72c 673a675 a5da72c 673a675 a5da72c 673a675 a5da72c 673a675 a5da72c 673a675 a5da72c 673a675 a5da72c 673a675 c2523f0 a5da72c 673a675 a5da72c 673a675 c2523f0 673a675 a5da72c 673a675 a5da72c 673a675 c2523f0 673a675 a5da72c 673a675 a5da72c 673a675 a5da72c 673a675 a5da72c 673a675 a5da72c 673a675 a5da72c 286159b |
1 2 3 4 5 6 7 8 9 10 11 12 13 14 15 16 17 18 19 20 21 22 23 24 25 26 27 28 29 30 31 32 33 34 35 36 37 38 39 40 41 42 43 44 45 46 47 48 49 50 51 52 53 54 55 56 57 58 59 60 61 62 63 64 65 66 67 68 69 70 71 72 73 74 75 76 77 78 79 80 81 82 83 84 85 86 87 88 89 90 91 92 93 94 95 96 97 98 99 100 101 102 103 104 105 106 107 108 109 110 111 112 113 114 115 116 117 118 119 120 121 122 123 124 125 126 127 128 129 130 131 132 133 134 135 136 137 138 139 140 141 142 143 144 145 146 147 148 149 150 151 152 153 154 155 156 157 158 159 160 161 162 163 164 165 166 167 168 169 170 171 172 173 174 175 176 177 178 179 180 181 182 183 184 185 186 187 188 189 190 191 192 193 194 195 196 197 198 199 200 201 202 203 204 205 206 207 208 209 210 211 212 213 214 215 216 217 218 219 220 221 222 223 224 225 226 227 228 229 230 231 232 233 234 235 236 237 238 239 240 241 242 243 244 245 246 247 248 249 250 251 252 253 254 255 256 257 258 259 260 261 262 263 264 265 266 267 268 269 270 271 272 273 274 275 276 277 278 279 280 281 282 283 284 285 286 287 288 289 290 291 292 293 294 295 296 297 298 299 300 301 302 303 304 305 306 307 308 309 310 311 312 313 314 315 316 317 318 319 320 321 322 323 324 325 326 327 328 329 330 331 332 333 334 335 336 337 338 339 340 341 342 343 344 345 346 347 348 349 350 351 352 353 |
---
sidebar_position: 7
slug: /deploy_local_llm
---
# Deploy a local LLM
import Tabs from '@theme/Tabs';
import TabItem from '@theme/TabItem';
Run models locally using Ollama, Xinference, or other frameworks.
RAGFlow supports deploying models locally using Ollama, Xinference, IPEX-LLM, or jina. If you have locally deployed models to leverage or wish to enable GPU or CUDA for inference acceleration, you can bind Ollama or Xinference into RAGFlow and use either of them as a local "server" for interacting with your local models.
RAGFlow seamlessly integrates with Ollama and Xinference, without the need for further environment configurations. You can use them to deploy two types of local models in RAGFlow: chat models and embedding models.
:::tip NOTE
This user guide does not intend to cover much of the installation or configuration details of Ollama or Xinference; its focus is on configurations inside RAGFlow. For the most current information, you may need to check out the official site of Ollama or Xinference.
:::
## Deploy local models using Ollama
[Ollama](https://github.com/ollama/ollama) enables you to run open-source large language models that you deployed locally. It bundles model weights, configurations, and data into a single package, defined by a Modelfile, and optimizes setup and configurations, including GPU usage.
:::note
- For information about downloading Ollama, see [here](https://github.com/ollama/ollama?tab=readme-ov-file#ollama).
- For information about configuring Ollama server, see [here](https://github.com/ollama/ollama/blob/main/docs/faq.md#how-do-i-configure-ollama-server).
- For a complete list of supported models and variants, see the [Ollama model library](https://ollama.com/library).
:::
### 1. Deploy ollama using docker
```bash
sudo docker run --name ollama -p 11434:11434 ollama/ollama
time=2024-12-02T02:20:21.360Z level=INFO source=routes.go:1248 msg="Listening on [::]:11434 (version 0.4.6)"
time=2024-12-02T02:20:21.360Z level=INFO source=common.go:49 msg="Dynamic LLM libraries" runners="[cpu cpu_avx cpu_avx2 cuda_v11 cuda_v12]"
```
Ensure ollama is listening on all IP address:
```bash
sudo ss -tunlp|grep 11434
tcp LISTEN 0 4096 0.0.0.0:11434 0.0.0.0:* users:(("docker-proxy",pid=794507,fd=4))
tcp LISTEN 0 4096 [::]:11434 [::]:* users:(("docker-proxy",pid=794513,fd=4))
```
Pull models as you need. It's recommended to start with `llama3.2` (a 3B chat model) and `bge-m3` (a 567M embedding model):
```bash
sudo docker exec ollama ollama pull llama3.2
pulling dde5aa3fc5ff... 100% ββββββββββββββββββ 2.0 GB
success
```
```bash
sudo docker exec ollama ollama pull bge-m3
pulling daec91ffb5dd... 100% ββββββββββββββββββ 1.2 GB
success
```
### 2. Ensure Ollama is accessible
If RAGFlow runs in Docker and Ollama runs on the same host machine, check if ollama is accessiable from inside the RAGFlow container:
```bash
sudo docker exec -it ragflow-server bash
root@8136b8c3e914:/ragflow# curl http://host.docker.internal:11434/
Ollama is running
```
If RAGFlow runs from source code and Ollama runs on the same host machine, check if ollama is accessiable from RAGFlow host machine:
```bash
curl http://localhost:11434/
Ollama is running
```
If RAGFlow and Ollama run on different machines, check if ollama is accessiable from RAGFlow host machine:
```bash
curl http://${IP_OF_OLLAMA_MACHINE}:11434/
Ollama is running
```
### 4. Add Ollama
In RAGFlow, click on your logo on the top right of the page **>** **Model Providers** and add Ollama to RAGFlow:

### 5. Complete basic Ollama settings
In the popup window, complete basic settings for Ollama:
1. Ensure model name and type match those been pulled at step 1, For example, (`llama3.2`, `chat`), (`bge-m3`, `embedding`).
2. Ensure that the base URL match which been determined at step 2.
3. OPTIONAL: Switch on the toggle under **Does it support Vision?** if your model includes an image-to-text model.
:::caution WARNING
Improper base URL settings will trigger the following error:
```bash
Max retries exceeded with url: /api/chat (Caused by NewConnectionError('<urllib3.connection.HTTPConnection object at 0xffff98b81ff0>: Failed to establish a new connection: [Errno 111] Connection refused'))
```
:::
### 6. Update System Model Settings
Click on your logo **>** **Model Providers** **>** **System Model Settings** to update your model:
*You should now be able to find **llama3.2** from the dropdown list under **Chat model**, and **bge-m3** from the dropdown list under **Embedding model**.*
> If your local model is an embedding model, you should find your local model under **Embedding model**.
### 7. Update Chat Configuration
Update your chat model accordingly in **Chat Configuration**:
> If your local model is an embedding model, update it on the configuration page of your knowledge base.
## Deploy a local model using Xinference
Xorbits Inference ([Xinference](https://github.com/xorbitsai/inference)) enables you to unleash the full potential of cutting-edge AI models.
:::note
- For information about installing Xinference Ollama, see [here](https://inference.readthedocs.io/en/latest/getting_started/).
- For a complete list of supported models, see the [Builtin Models](https://inference.readthedocs.io/en/latest/models/builtin/).
:::
To deploy a local model, e.g., **Mistral**, using Xinference:
### 1. Check firewall settings
Ensure that your host machine's firewall allows inbound connections on port 9997.
### 2. Start an Xinference instance
```bash
$ xinference-local --host 0.0.0.0 --port 9997
```
### 3. Launch your local model
Launch your local model (**Mistral**), ensuring that you replace `${quantization}` with your chosen quantization method:
```bash
$ xinference launch -u mistral --model-name mistral-v0.1 --size-in-billions 7 --model-format pytorch --quantization ${quantization}
```
### 4. Add Xinference
In RAGFlow, click on your logo on the top right of the page **>** **Model Providers** and add Xinference to RAGFlow:

### 5. Complete basic Xinference settings
Enter an accessible base URL, such as `http://<your-xinference-endpoint-domain>:9997/v1`.
> For rerank model, please use the `http://<your-xinference-endpoint-domain>:9997/v1/rerank` as the base URL.
### 6. Update System Model Settings
Click on your logo **>** **Model Providers** **>** **System Model Settings** to update your model.
*You should now be able to find **mistral** from the dropdown list under **Chat model**.*
> If your local model is an embedding model, you should find your local model under **Embedding model**.
### 7. Update Chat Configuration
Update your chat model accordingly in **Chat Configuration**:
> If your local model is an embedding model, update it on the configuration page of your knowledge base.
## Deploy a local model using IPEX-LLM
[IPEX-LLM](https://github.com/intel-analytics/ipex-llm) is a PyTorch library for running LLMs on local Intel CPUs or GPUs (including iGPU or discrete GPUs like Arc, Flex, and Max) with low latency. It supports Ollama on Linux and Windows systems.
To deploy a local model, e.g., **Qwen2**, using IPEX-LLM-accelerated Ollama:
### 1. Check firewall settings
Ensure that your host machine's firewall allows inbound connections on port 11434. For example:
```bash
sudo ufw allow 11434/tcp
```
### 2. Launch Ollama service using IPEX-LLM
#### 2.1 Install IPEX-LLM for Ollama
:::tip NOTE
IPEX-LLM's supports Ollama on Linux and Windows systems.
:::
For detailed information about installing IPEX-LLM for Ollama, see [Run llama.cpp with IPEX-LLM on Intel GPU Guide](https://github.com/intel-analytics/ipex-llm/blob/main/docs/mddocs/Quickstart/llama_cpp_quickstart.md):
- [Prerequisites](https://github.com/intel-analytics/ipex-llm/blob/main/docs/mddocs/Quickstart/llama_cpp_quickstart.md#0-prerequisites)
- [Install IPEX-LLM cpp with Ollama binaries](https://github.com/intel-analytics/ipex-llm/blob/main/docs/mddocs/Quickstart/llama_cpp_quickstart.md#1-install-ipex-llm-for-llamacpp)
*After the installation, you should have created a Conda environment, e.g., `llm-cpp`, for running Ollama commands with IPEX-LLM.*
#### 2.2 Initialize Ollama
1. Activate the `llm-cpp` Conda environment and initialize Ollama:
<Tabs
defaultValue="linux"
values={[
{label: 'Linux', value: 'linux'},
{label: 'Windows', value: 'windows'},
]}>
<TabItem value="linux">
```bash
conda activate llm-cpp
init-ollama
```
</TabItem>
<TabItem value="windows">
Run these commands with *administrator privileges in Miniforge Prompt*:
```cmd
conda activate llm-cpp
init-ollama.bat
```
</TabItem>
</Tabs>
2. If the installed `ipex-llm[cpp]` requires an upgrade to the Ollama binary files, remove the old binary files and reinitialize Ollama using `init-ollama` (Linux) or `init-ollama.bat` (Windows).
*A symbolic link to Ollama appears in your current directory, and you can use this executable file following standard Ollama commands.*
#### 2.3 Launch Ollama service
1. Set the environment variable `OLLAMA_NUM_GPU` to `999` to ensure that all layers of your model run on the Intel GPU; otherwise, some layers may default to CPU.
2. For optimal performance on Intel Arcβ’ A-Series Graphics with Linux OS (Kernel 6.2), set the following environment variable before launching the Ollama service:
```bash
export SYCL_PI_LEVEL_ZERO_USE_IMMEDIATE_COMMANDLISTS=1
```
3. Launch the Ollama service:
<Tabs
defaultValue="linux"
values={[
{label: 'Linux', value: 'linux'},
{label: 'Windows', value: 'windows'},
]}>
<TabItem value="linux">
```bash
export OLLAMA_NUM_GPU=999
export no_proxy=localhost,127.0.0.1
export ZES_ENABLE_SYSMAN=1
source /opt/intel/oneapi/setvars.sh
export SYCL_CACHE_PERSISTENT=1
./ollama serve
```
</TabItem>
<TabItem value="windows">
Run the following command *in Miniforge Prompt*:
```cmd
set OLLAMA_NUM_GPU=999
set no_proxy=localhost,127.0.0.1
set ZES_ENABLE_SYSMAN=1
set SYCL_CACHE_PERSISTENT=1
ollama serve
```
</TabItem>
</Tabs>
:::tip NOTE
To enable the Ollama service to accept connections from all IP addresses, use `OLLAMA_HOST=0.0.0.0 ./ollama serve` rather than simply `./ollama serve`.
:::
*The console displays messages similar to the following:*
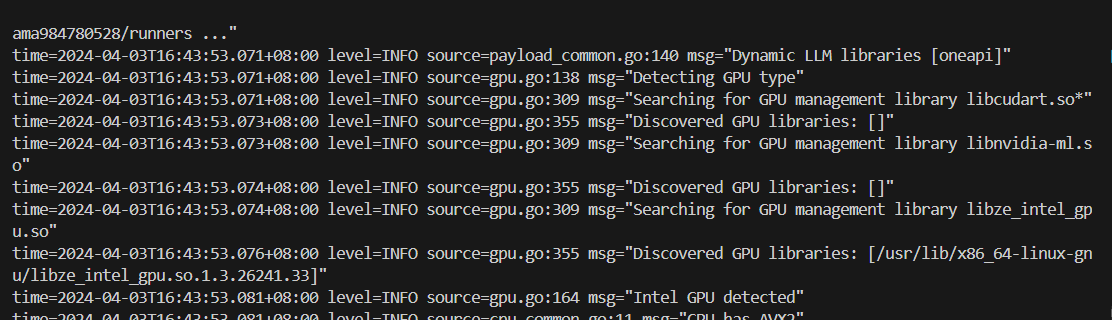
### 3. Pull and Run Ollama model
#### 3.1 Pull Ollama model
With the Ollama service running, open a new terminal and run `./ollama pull <model_name>` (Linux) or `ollama.exe pull <model_name>` (Windows) to pull the desired model. e.g., `qwen2:latest`:
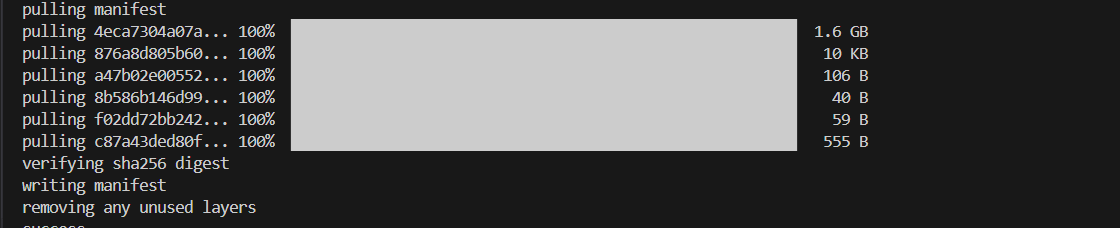
#### 3.2 Run Ollama model
<Tabs
defaultValue="linux"
values={[
{label: 'Linux', value: 'linux'},
{label: 'Windows', value: 'windows'},
]}>
<TabItem value="linux">
```bash
./ollama run qwen2:latest
```
</TabItem>
<TabItem value="windows">
```cmd
ollama run qwen2:latest
```
</TabItem>
</Tabs>
### 4. Configure RAGflow
To enable IPEX-LLM accelerated Ollama in RAGFlow, you must also complete the configurations in RAGFlow. The steps are identical to those outlined in the *Deploy a local model using Ollama* section:
1. [Add Ollama](#4-add-ollama)
2. [Complete basic Ollama settings](#5-complete-basic-ollama-settings)
3. [Update System Model Settings](#6-update-system-model-settings)
4. [Update Chat Configuration](#7-update-chat-configuration)
## Deploy a local model using jina
To deploy a local model, e.g., **gpt2**, using jina:
### 1. Check firewall settings
Ensure that your host machine's firewall allows inbound connections on port 12345.
```bash
sudo ufw allow 12345/tcp
```
### 2. Install jina package
```bash
pip install jina
```
### 3. Deploy a local model
Step 1: Navigate to the **rag/svr** directory.
```bash
cd rag/svr
```
Step 2: Run **jina_server.py**, specifying either the model's name or its local directory:
```bash
python jina_server.py --model_name gpt2
```
> The script only supports models downloaded from Hugging Face. |