File size: 6,219 Bytes
8dc9a1e |
1 2 3 4 5 6 7 8 9 10 11 12 13 14 15 16 17 18 19 20 21 22 23 24 25 26 27 28 29 30 31 32 33 34 35 36 37 38 39 40 41 42 43 44 45 46 47 48 49 50 51 52 53 54 55 56 57 58 59 60 61 62 63 64 65 66 67 68 69 70 71 72 73 74 75 76 77 78 79 80 81 82 83 84 85 86 87 88 89 90 91 92 93 94 95 96 97 98 99 100 101 102 103 104 105 106 107 108 109 110 111 112 113 114 115 116 117 118 119 120 121 122 123 124 125 126 127 128 129 130 131 |
# Sentiment Tuning Examples
The notebooks and scripts in this examples show how to fine-tune a model with a sentiment classifier (such as `lvwerra/distilbert-imdb`).
Here's an overview of the notebooks and scripts in the [trl repository](https://github.com/huggingface/trl/tree/main/examples):
| File | Description |
|------------------------------------------------------------------------------------------------------------|--------------------------------------------------------------------------------------------------------------------------|
| [`examples/scripts/ppo.py`](https://github.com/huggingface/trl/blob/main/examples/scripts/ppo.py) [](https://colab.research.google.com/github/huggingface/trl/blob/main/examples/sentiment/notebooks/gpt2-sentiment.ipynb) | This script shows how to use the `PPOTrainer` to fine-tune a sentiment analysis model using IMDB dataset |
| [`examples/notebooks/gpt2-sentiment.ipynb`](https://github.com/huggingface/trl/tree/main/examples/notebooks/gpt2-sentiment.ipynb) | This notebook demonstrates how to reproduce the GPT2 imdb sentiment tuning example on a jupyter notebook. |
| [`examples/notebooks/gpt2-control.ipynb`](https://github.com/huggingface/trl/tree/main/examples/notebooks/gpt2-control.ipynb) [](https://colab.research.google.com/github/huggingface/trl/blob/main/examples/sentiment/notebooks/gpt2-sentiment-control.ipynb) | This notebook demonstrates how to reproduce the GPT2 sentiment control example on a jupyter notebook.
## Usage
```bash
# 1. run directly
python examples/scripts/ppo.py
# 2. run via `accelerate` (recommended), enabling more features (e.g., multiple GPUs, deepspeed)
accelerate config # will prompt you to define the training configuration
accelerate launch examples/scripts/ppo.py # launches training
# 3. get help text and documentation
python examples/scripts/ppo.py --help
# 4. configure logging with wandb and, say, mini_batch_size=1 and gradient_accumulation_steps=16
python examples/scripts/ppo.py --log_with wandb --mini_batch_size 1 --gradient_accumulation_steps 16
```
Note: if you don't want to log with `wandb` remove `log_with="wandb"` in the scripts/notebooks. You can also replace it with your favourite experiment tracker that's [supported by `accelerate`](https://huggingface.co/docs/accelerate/usage_guides/tracking).
## Few notes on multi-GPU
To run in multi-GPU setup with DDP (distributed Data Parallel) change the `device_map` value to `device_map={"": Accelerator().process_index}` and make sure to run your script with `accelerate launch yourscript.py`. If you want to apply naive pipeline parallelism you can use `device_map="auto"`.
## Benchmarks
Below are some benchmark results for `examples/scripts/ppo.py`. To reproduce locally, please check out the `--command` arguments below.
```bash
python benchmark/benchmark.py \
--command "python examples/scripts/ppo.py --log_with wandb" \
--num-seeds 5 \
--start-seed 1 \
--workers 10 \
--slurm-nodes 1 \
--slurm-gpus-per-task 1 \
--slurm-ntasks 1 \
--slurm-total-cpus 12 \
--slurm-template-path benchmark/trl.slurm_template
```
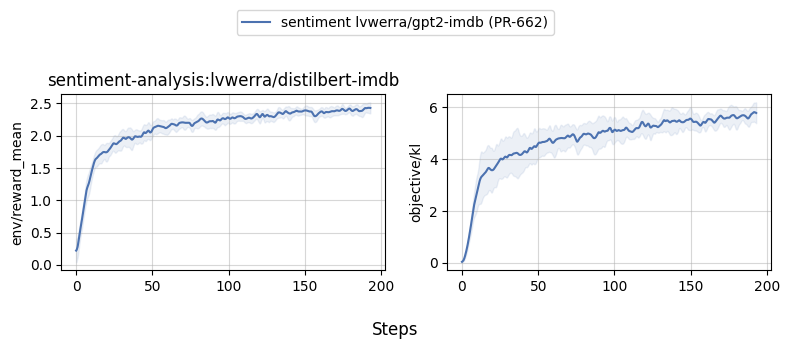
## With and without gradient accumulation
```bash
python benchmark/benchmark.py \
--command "python examples/scripts/ppo.py --exp_name sentiment_tuning_step_grad_accu --mini_batch_size 1 --gradient_accumulation_steps 128 --log_with wandb" \
--num-seeds 5 \
--start-seed 1 \
--workers 10 \
--slurm-nodes 1 \
--slurm-gpus-per-task 1 \
--slurm-ntasks 1 \
--slurm-total-cpus 12 \
--slurm-template-path benchmark/trl.slurm_template
```
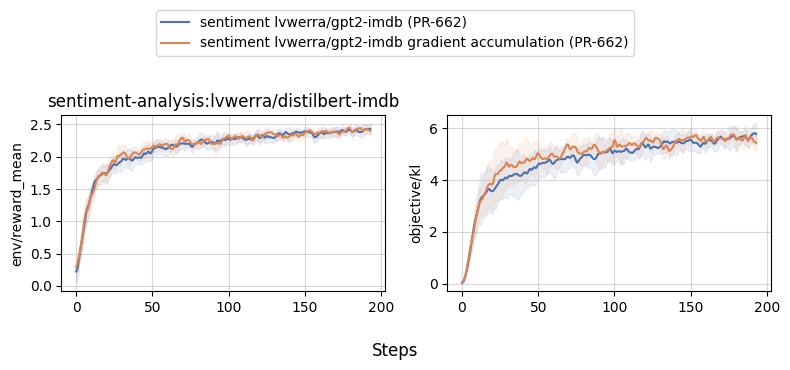
## Comparing different models (gpt2, gpt2-xl, falcon, llama2)
```bash
python benchmark/benchmark.py \
--command "python examples/scripts/ppo.py --exp_name sentiment_tuning_gpt2 --log_with wandb" \
--num-seeds 5 \
--start-seed 1 \
--workers 10 \
--slurm-nodes 1 \
--slurm-gpus-per-task 1 \
--slurm-ntasks 1 \
--slurm-total-cpus 12 \
--slurm-template-path benchmark/trl.slurm_template
python benchmark/benchmark.py \
--command "python examples/scripts/ppo.py --exp_name sentiment_tuning_gpt2xl_grad_accu --model_name gpt2-xl --mini_batch_size 16 --gradient_accumulation_steps 8 --log_with wandb" \
--num-seeds 5 \
--start-seed 1 \
--workers 10 \
--slurm-nodes 1 \
--slurm-gpus-per-task 1 \
--slurm-ntasks 1 \
--slurm-total-cpus 12 \
--slurm-template-path benchmark/trl.slurm_template
python benchmark/benchmark.py \
--command "python examples/scripts/ppo.py --exp_name sentiment_tuning_falcon_rw_1b --model_name tiiuae/falcon-rw-1b --log_with wandb" \
--num-seeds 5 \
--start-seed 1 \
--workers 10 \
--slurm-nodes 1 \
--slurm-gpus-per-task 1 \
--slurm-ntasks 1 \
--slurm-total-cpus 12 \
--slurm-template-path benchmark/trl.slurm_template
```
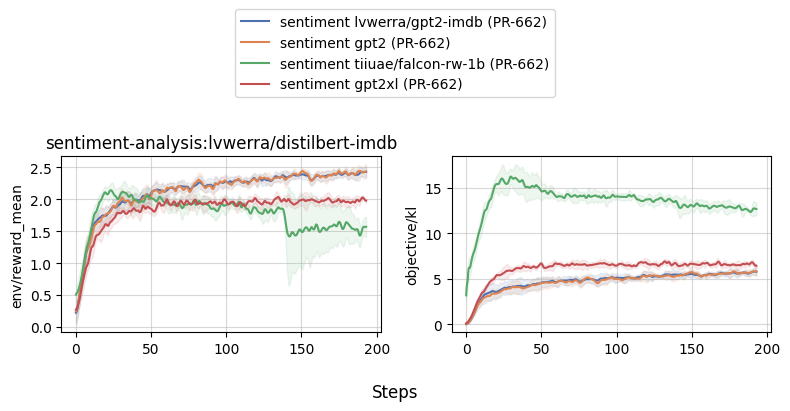
## With and without PEFT
```
python benchmark/benchmark.py \
--command "python examples/scripts/ppo.py --exp_name sentiment_tuning_peft --use_peft --log_with wandb" \
--num-seeds 5 \
--start-seed 1 \
--workers 10 \
--slurm-nodes 1 \
--slurm-gpus-per-task 1 \
--slurm-ntasks 1 \
--slurm-total-cpus 12 \
--slurm-template-path benchmark/trl.slurm_template
```
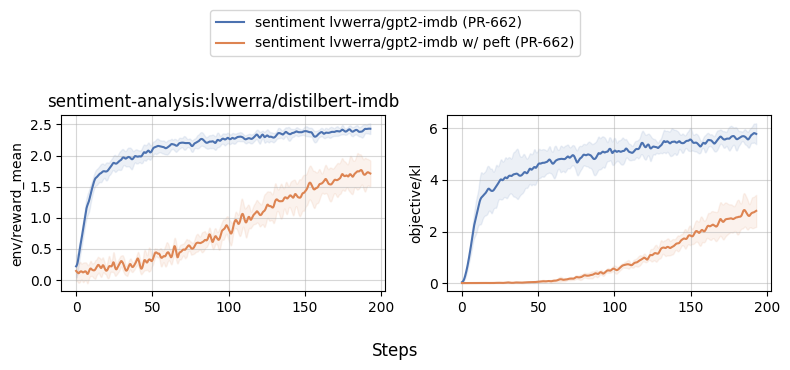
|